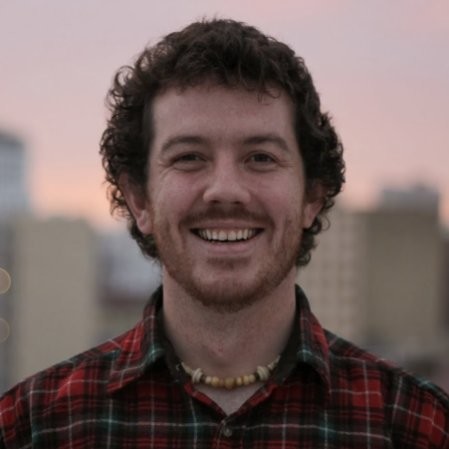
We're pretty proud of our backend test suite. We have a lot of tests, and developers can run the full test suite locally in under six minutes. These aren't simple unit tests—they're tests that hit multiple databases and routes with only a minimal amount of stubbing for external dependencies.
9 years ago, we were proud of running 2,000 tests in 3 minutes. Not much has changed from that initial post—we're still writing a bunch of tests, but we've put a lot of effort over the years into making sure our test suite has stayed acceptably fast for people.
Why do we run our tests this way?
First off though, why are we making things so hard for ourselves? When we write our tests, we don't stub out our databases at all. Many of our tests are resource tests—those tests hit a real running server, the resource code issues real queries against Redis/MySQL/MongoDB/memcached containers, and if it makes any changes to those databases, we need to reset the databases fully before the next test run.
We think that the database is an integral part of the system that we're testing. When you stub a database query, that means that you're not testing the query. And I don't know about you, but I've gotten plenty of database queries wrong.
Similarly, we like to run a full server for any resource level tests. The middleware that runs for each resource matters. We want our tests to match our production environment as much as possible.
How do we make the tests fast?
You'll see recommendations online to limit this style of testing, where you have full databases that you're querying, not because it's worse, but because it ends up being too slow. We've needed to put a lot of work into test speed over the years, and if you take nothing else away from this post it should be that if you treat test speed as an organizational priority, you can make a pretty big impact.
-
Make sure engineers have fast computers. First off, if we had done nothing else over the past 9 years, our tests would have gotten faster because computers have gotten better over that time period. And we make sure to buy nice computers for engineers on our team because we care that tests and builds are speedy. (The M1 and M2 chips for Macs have been quite nice!)
-
Use Orbstack rather than Docker Desktop. The single easiest change to speed up our tests was switching from Docker Desktop to Orbstack to run containers locally. On Macs, it is so much faster than Docker Desktop. On some engineers' machines, tests run twice as fast on Orbstack. Aside from speed, we've found that it's been more stable for folks—fewer randomly missing volumes and needing to restart Docker to get things going again. We're huge fans.
That said though, it's worth noting using docker/orbstack will still slow down your tests. If we ran databases directly on our machines rather than through docker, our tests would be faster. But the extra effort to get everyone to install and maintain MySQL, Redis, MongoDB, Memcached, and everything else just isn't worth the test speed increases that it brings for us. Other organizations might have different trade-offs.
-
Speed up fixture resets. The slowest part of our tests is resetting fixtures. Whenever one of our tests writes to a database, we need to undo those changes before the next test starts. The core trick to doing this quickly is to only undo changes to the tables that actually changed rather than resetting every single table. All of our database operations go through the same code, so it's relatively straightforward to track which tables are "dirty" and then only reset those tables.
A few details:
- We tested out tracking things at the row level rather than the table level for resets, but it didn't improve performance. For MySQL, our basic table resetting strategy is turning off foreign key checks, truncating the table, and then using
LOCAL DATA INFILE
loads from a volume that's mounted into the MySQL container. - For MongoDB resets, we found that the fastest technique was creating a shadow collection for every collection that we could restore from whenever we needed to.
- When there's a hard MySQL delete, we don't know whether it might be a cascading delete, so we have code that counts how many rows are in each table. If a table has fewer rows after the test we should reset it. And if it doesn't have fewer rows because data has also been inserted, our regular code will have marked that table as dirty.
- For MySQL updates, we have some (slightly janky) code to pull out the list of tables that might be updated by the update query when it's a query with multiple tables.
- We tested out tracking things at the row level rather than the table level for resets, but it didn't improve performance. For MySQL, our basic table resetting strategy is turning off foreign key checks, truncating the table, and then using
-
Run tests in parallel. The next important piece to fast tests is being able to run the test suite in parallel, which means we need multiple copies of our databases. This was a relatively straightforward task that took a lot of blood, sweat, and tears to actually make happen. We use
mocha
to run our tests which supports a--parallel
option, so our tests look forMOCHA_WORKER_ID
in the environment to decide which database to connect to:test_db_${MOCHA_WORKER_ID}
. -
Measure what's slow. Like any other optimization problem, the first step is measuring how long things actually take. Having guesses about why tests are slow can lead to a ton of wasted effort that doesn't actually move the needle. We haven't done any fancy profiling here—instead, we hook into our existing instrumentation to generate a report of where time is being spent over the course of our tests. It's not perfect, but it gives us a good enough sense of where time is going to be useful.
The future
We're proud of where our tests are, but there's still a ton of space to improve things over the next 9 years. We're going to keep writing lots of tests, so we need to make sure that those tests continue to be speedy. A few things that are on our minds:
- Setting up fixture "scenarios." Currently, we always reset our fixtures back to the same base scenario. That base scenario is difficult to change because it can impact a huge number of tests if we're tweaking fixture data that is used in a lot of spots, so it'd be nice to have better support for temporarily setting up a new "base" state that multiple tests can reference.
- Retrying the row-level resets. In theory, these should be faster than truncating and restoring the tables, so we want to try out better profiling to make that happen.
- Improving our Redis fixture resets. Redis is fast enough that we've been lazy with our Redis fixture resets—we just flush the db and then restore it, so there's room to improve performance
- Run our tests with some more detailed profiling to generate a flame-graph to see if there are any hotspots in our code that we could improve. Funnily enough, we've actually sped up our production app a few times when optimizing our tests—things that are slow in testing are often slow in production too!