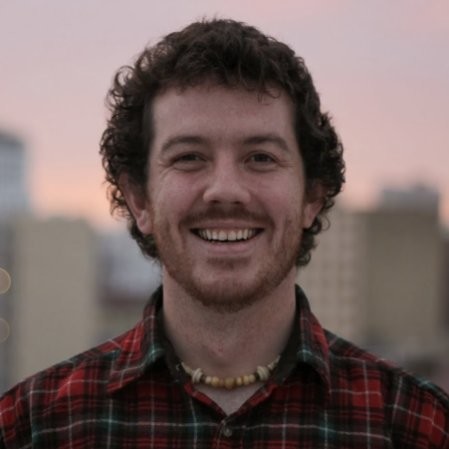
Years ago, I wanted to update a bad pattern in our codebase—updating the order of arguments to a function or something like that. This particular pattern was only in about 10 spots, so it would have only taken a minute to search and fix manually, but instead I spent an hour automating the fix using sed
and xargs
. And I think that was the right choice.
At the time, I had no clue what I was doing in the shell. sed
confused me, shell quoting rules were totally opaque, and xargs
was a black box. I remember feeling so stupid because I couldn't figure out how to automate this reoroder; it was the kind of thing that should be simple, but I kept getting stuck.
But I automated that refactor, and I learned a few things! I learned that the default sed
on Macs was quite old, that -r
is necessary for regular expression mode, how to use capture groups, how to use -i
with gsed
, the difference between '
and "
in the shell, how to use xargs
with gsed -i
, and probably a few other things. If I'd found better resources, I could have learned those things faster, but being comfortable with shell tools has saved me so much time over the years when I've run into situations that aren't fixable manually.[^1]
Just a few days ago, I wanted to stitch together 15 markdown documents, format them in a nice-ish way, and then print them. The easy way would have been to copy-paste 15 times. It probably would have taken a minute. Instead, I spent a chunk of time writing a python script to do it for me, and I learned a few more things. I somehow hadn't put together that you can just write html in markdown and it just works because it's left alone during the conversion process. That seems glaringly obvious in retrospect, but I'm glad I learned it. I also learned pandoc is great for converting markdown to html, this CSS from killercup works great with pandoc --css pandoc.css --standalone
to make the pandoc-converted html prettier, figured out where my Obsidian Vault was stored on disk, and learned the CSS to make nice line-breaks (<div style="page-break-after: always;"></div>
) between the 15 markdown documents. Doing the work to automate stitching together these 15 markdown documents was far slower than copy-pasting them, but over the long-term, automating builds compounding skills.
I'll sometimes see people reference XKCD's Is It Worth the Time to argue against automating things that would be faster to do manually, and I think that that mindset is a mistake. Automating the easy things is how you build the skills, mindset, and muscle-memory to automate the hard things. There are situations where the only thing that matters is that a task gets accomplished quickly; in those cases, doing it manually can make sense! But a lot of the time, trying to automate the thing builds skill and capability that will come in handy later.
Aside from building personal capability, reaching for automation is an important part of building an engineering culture that values automation. An engineering culture that values automation is going to find opportunities to reduce toil and speed through projects that another engineering culture might miss. I'd like to have an engineering culture that celebrates the times when the team learned how to automate something, even when automating the thing took much longer than just doing the thing. The accumulation of capability trumps the loss in short-term speed.
So, the next time you want to rewrite the order of arguments in a function that's only used in three spots in a codebase, spend some time figuring out regular expressions instead. The next time you realize you forgot to run a command that's necessary for something, spend an unreasonable amount of effort making it so that the command runs automatically. The next time you have something you need to only do yearly, waste some time building tooling to make it easier for yourself.
[^1]: ag -l doThing | xargs gsed -r 's|doThing\(([^,]+), ([^)]+)\)|doThing(\2, \1)|g'
is a reasonable way of re-ordering a function's arguments (Bash Patterns I Use Weekly describes how this command works). Code editors should have this built in to their find-and-replace, but I prefer doing it on the shell because it allows me to construct more complex commands that aren't possible with a GUI.