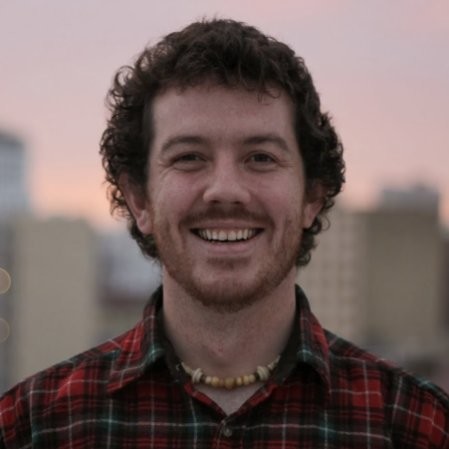
A single large query in SQL can be hard to understand, test, debug, or change in the same way that an over-large function in code can be. A large query is also much harder to write! Feedback loops while writing large queries are slow and you'll often find yourself needing to guess at where the problem in your query is.
When I started writing analytics queries, I wrote some pretty rough ones that are now hard to debug and maintain! (Apologies to everyone who has had to deal with any of my old SQL queries.) Over time, I think I've gotten better at them, and I wanted to write down some of the things I'm doing differently now that I hope will be useful to other people in similar situations.
Finally, I'm a product engineer who rarely writes these sorts of queries! This advice is much less applicable to someone who does this daily & is using better & more focused tools to do analyses.
Temporary tables, views, and with
Large queries are hard, but it's pretty simple to break a large query into smaller pieces. For analytics queries, I normally create small temporary tables (often with the temporary
keyword) that normalize data, filter out deleted rows and rows I'm not interested in, and organize my data into a format that makes querying easy. Views or with
clauses can accomplish similar things, but I like using temporary tables for this because they cache results and make subsequent queries faster.
I also try to put constants into a temporary table or with
clause. When working on a query, it can be easy to forget to update a constant in one spot and then get completely meaningless results. (Shoutout to Ben Haley for showing me this trick!)
All of this might sound a little abstract: let's take a somewhat contrived query and try to refactor it. We want to bucket and count US-based teachers who were active in 2021 by how many classes they created during that time period. Here's what that might look like as a single query:
select
case when class_count < 5 then class_count::varchar else 'many' end as bucket,
count(*)
from (
select count(distinct class.classId) as class_count
from teacher
join user_teacher ON teacher.teacherId = user_teacher.teacherid
-- left join class_teacher to make sure we're counting teachers who haven't created classes
left join class_teacher on class_teacher.teacherId = user_teacher.teacherId and class_teacher.creator
left join user USING(userId)
join class using(classId)
join (
select distinct teacherId
from teacher_active
where active_date between '2021-01-01' and '2022-01-01'
) as ats on teacher.teacherId = ats.teacherId
and class.createdat between '2021-01-01' and '2022-01-01'
and not class.autocreated_demo
and lower(user.country) in ('usa', 'us')
group by teacherId
)
group by 1
This query isn't particularly complex, but it's still enough logic that I'd be a little worried about changing it or verifying that it's correct. I'd be tempted to try to pull out constants and then separate out the filtering logic from the calculation logic.
drop table if exists _constant;
create temporary table _constant as (
select '2021-01-01' as start, '2022-01-01' as end
);
drop table if exists _teacher;
create temporary table _teacher as (
-- us_user is probably overkill: this might be better in the `where` clause!
with us_user as (
select userId
from user
where lower(country) in ('usa', 'us')
)
select distinct teacherId
from teacher_active
join user_teacher USING(teacherId)
join us_user using(userid)
where active_date between (select start from _constant)
and (select end from _constant)
);
drop table if exists _class;
create temporary table _class (
select classId
from class
where class.created between (select start from _constant)
and (select end from _constant)
and not class.autocreated_demo
);
drop table if exists _classes_created_by_teacher;
create temporary table _classes_created_by_teacher (
with class_creator as (
select class_teacher.*
from class_teacher
join _class USING(classId)
where class_teacher.creator
)
select teacherId, count(distinct classId) as classes_created
from _teacher
left join class_creator using(teacherId)
group by teacherId
);
select
case when class_count < 5 then class_count::varchar else 'many' end as bucket,
count(*)
from _classes_created_by_teacher
group by bucket;
It's arguable whether this is actually better! The initial query is short enough that it's not that much logic to understand: it might be the right size for the team that you're working with. There are also certainly better ways of factoring this same query that could make the logic even more clear. Overall though, I'd much rather work with the updated query:
- if something is broken in the query, I can easily and quickly examine the tables that I've created to see if my results match my expectations
- if I have a testing library of some sort, I can set up simple assertions about what the tables I'm using look like
- the overall query will run faster because results are cached and because query-planners don't always generate optimum plans for large queries. While working on this query, I'll have faster feedback loops
- I'll be able to tell which parts of this query are slow and optimize if necessary
- it's easier to focus on adapting & improving a single part
- The sub-queries that I've turned into tables are things I could take advantage of later if I ever tackle other similar problems by turning them into nice non-temporary cache tables
I think many data-focused engineers use jupyter
notebooks and pandas
to break down large queries. I think how you're breaking down a large query into smaller pieces is much less important than doing that breakdown!
Make feedback loops FAST!
One of the most frustrating parts of working on a large query is that feedback loops can be slow. Making a change and waiting tens of minutes can completely kill any programming flow or focus that you have.
- Break up large queries into smaller ones that are quick to run!
- Use
select
without afrom
to quickly test out behavior. You can run queries likeselect extract('year' from GETDATE());
orselect 1 != null, 1 is not null
to quickly check your understanding. This can be especially useful for testing out regular expressions and formatting date fields for charts. There's no need for afrom
or to run a full query1 - If a full table is slow to query, it might make sense to create a temporary table with representative data that you can use to build up your queries
- Good indexes or sort-keys can drastically improve query speed! The most common mistake I've made is setting up a compound sort key with a too-precise timestamp followed by other keys I'm interested in. If you use a second or millisecond precision key at the start of a compound sortkey, that key's precision will override any possible benefit from the other key. So, rather than creating a sortkey like
(createdAtMs, event)
, it's probably better to instead sort and query on an index like(day, event)
. (This might mean that queries need to include bothday
andcreatedAtMs
.) - validate your work as you go! The quicker you can realize that your assumptions about a table or column are incorrect the better. Running a query that checks whether a column is unique, what values a column can hold, or just what data looks like can save a ton of time!
In general, putting effort into how quickly you get feedback while working makes it much easier to find flow and be effective. A little bit of effort put into setting up nice tables, improving data layout, and optimizing sortkeys can pay large dividends.